Creatively Disrupting the Intelligence Paradigm
13 Aug 2014
By Gregory Treverton for ISN
The traditional intelligence cycle is very linear and resembles a production line.[1] Portrayed in figure 1 in something of a caricature, it proceeds from requirements, to collection, to processing and analysis, to dissemination. Although in reality steps are skipped, and the process is nowhere near as tidy as the diagram suggests, its logic remains. That logic, however, is creatively disrupted by the most important innovation in intelligence analysis to come out of the wars in Iraq and Afghanistan – what’s called “activity-based intelligence,” or ABI. More fully embracing the principles of ABI can help to overcome the pitfalls of traditional intelligence and to meet the intelligence needs of the future.
Figure 1: Traditional Intelligence Cycle [2]
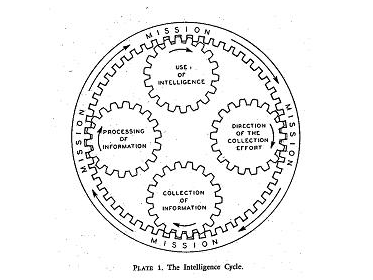
ABI vs. traditional intelligence
ABI was driven by the change in intelligence target, from nations we knew to terrorists we didn’t. It was going after what Donald Rumsfeld called the “unknown unknowns,” things we didn’t know we didn’t know.[3] As one of the creators of ABI put the change with regard to imagery: we used to know what we were looking for and be looking for things; now we don’t know what we’re looking for, and we’re not looking for things but rather activities. The targets of the fight against terror are small, not big; adaptive, not ponderous; and, perhaps most important, lack both signature and doctrine. A Soviet T-72 tank had a distinctive signature, one easily captured by a single intelligence discipline, imagery or IMINT, and was embedded in doctrine: if one was seen, a given number of its brothers could be expected as well. The intelligence process was linear and inductive, reasoning from a single sighting to a larger category of phenomena. By contrast, no predictable or semi-permanent signature distinguished the would-be implanters of IEDs from ordinary pious Muslims at mosques. Even if a cell phone signature could be resolved to a suspected terrorist, the next day could easily see a different phone entirely.
ABI surely will find uses beyond the battlefields of the Middle East, in distinguishing bad guys from good on the sea, in law enforcement or cyberspace. Perhaps more important, though, comparing the traditional cycle with ABI underscores how much the “cycle” enshrines a whole series of assumptions that are unhelpful and unwise. For starters, it assumes that we know what we’re looking for. The process begins with “requirements.” By contrast, ABI assumes we don’t know what we’re looking for. The cycle also assumes linearity. Sure, steps are skipped, but the basic model is linear. That sequence also assumes that what is disseminated is a “product”; it tends to turn intelligence into a commodity. Even the best writing on intelligence, like Roberta Wohlstetter’s book on World War II, through the wonderfully written 9/11 report, is linear: “dots” were there, if only they had been connected.
But think about how non-linear most thought actually is, and surely most of creativity. This is what, in ABI, is known as sequence neutrality. In intelligence, as in life, we often may solve a puzzle before we realize the puzzle was in our minds. Or think of the analogy with medicine, notice how many drugs were discovered by accident to be effective for one condition when they were being used for something else entirely. The discovery was an accident, hardly the result of a linear production function.
Perhaps most important and least helpful, traditional intelligence gives pride of place to collection. Collection drives the entire process: there is nothing to analyze if nothing is collected against the requirements that have been identified. When analysis or postmortems reveal an intelligence “gap,” the first response is to collect more data to fill that gap. But the world is full of data. Any “collection” is bound to be very selective and very partial. Perhaps slightly paradoxically, ABI reminds us that data are not really the problem. Data are everywhere, and getting more so, whether we like it or not. By one estimate, China has one camera for every 43 people. Privacy, as it was traditionally conceived, is either gone or about to be, again for better or worse.
Not only does traditional intelligence privilege collection, it still privileges the information it collects, often from its special or secret sources. The founding fathers of intelligence in the United States (alas, there were few mothers aside from Roberta Wohlstetter) worried, as Kent put it, that the concentration on clandestine collection would deform open collection. And so it has, in spades. For ABI, by contrast, data is neutral. There are no “reliable sources”—or questionable ones. Data only become “good” or “bad” when used. This neutrality of data is, not surprisingly, perhaps the most uncomfortable challenge of ABI to the traditional paradigm of intelligence, for so much of the cycle has been built around precisely the opposite—evaluating information sources for their reliability.
ABI turns on correlation: looking at patterns of activity to determine patterns of life and so networks. It is agnostic about causation, looking first for how things fit together. But the idea of correlation also opens up traditional analysis, for the linear process can all too easily lead to linear thinking—“drivers,” after all, imply causation. But causation in human affairs is a very complicated affair. Imagine if analysts instead grew up in a world of correlations—indicators—rather than drivers that impute causation where none usually obtains. They might be attuned to connections, perhaps even ones not natural to analysis, or to life. The idea that a street vendor in Tunisia who set himself on fire might in turn propel activists in Egypt to action surely wouldn’t occur but might be less easily dismissed out of hand.
So imagine a “might have been” and a “might be.” With regard to the Arab spring, it was hardly a secret that the countries involved were unstable. A generation of work by the CIA, other services, and their academic counterparts had created indicators of political instabilities, which were then turned into countries to watch. Those lists tended to be noted by policy officers but were never found very helpful because they could not specify when or how explosions might happen. The point was a fair one. From the perspective of traditional intelligence, the idea that a street vendor setting himself on fire in Tunisia could lead to uprising in a half dozen other countries was a black swan indeed. Had anyone suggested it, the suggestion would have fallen in the “outrageous” category. It would not – perhaps could not – have been recognized as the answer to another puzzle that had yet to be framed. [4]
But what if analysts had been looking for correlations, not causation? Suppose they had been monitoring Twitter or other open sources, and laying them against other sources both open and secret. The process could hardly have been definitive. But it might have provided hints, or allowed analysts to suggest other things to look at. At a minimum, it might have helped to weaken the power of mindset—in this case, the mindset that nothing in Tunisia could influence what happened in Egypt – by keeping the focus on data.
New places to look
In the category of “might be,” consider China’s future. It has long been assumed that, sooner or later, growth would slow and that the social change driven by economic growth would lead to political change.[5] Now, there is a good argument, though hardly proof, that the timing is “sooner.” Demographics is one powerful factor. China will soon fall off the demographic cliff, as the fruits of the one-child policy mean a rapidly aging population. And critically, China will begin to age when it is still relatively poor, and thus growing old for the Chinese will not be like aging in Japan or Europe.
Moreover, for both economic growth and political change, the key indicator is for China to reach a per capita income of $17,000 per year in 2005 purchasing power parity.[6] That will occur in 2015 if China continues to grow at 9–10 percent annually, or in 2017 if growth slows to 7 percent. Again, correlations are not predictions, but no non-oil-rich country with a per capita income over that level is ranked as other than “free” or “partly free” by Freedom House, while China is now deep in the “non-free” category.[7] As China has gotten richer, its people have become less inhibited in raising their voices, and the Internet provides them increasing opportunities, notwithstanding the government’s zeal in censoring it. The government has stopped enumerating what it calls “mass incidents”—strikes or protests usually carefully crafted to stick to local issues—but an estimate for 2010 puts these at 160,000, up from 10,000 in 1995. Another indicator is the visible nervousness of China’s leaders about the polity over which they rule.
On the economic side, one recent study found that high growth in non-oil exporting countries ended at a per capita income of about $17,000. [8] There are good economic arguments for the threshold: the payoff for shifting workers from agriculture to industry diminishes, as does the benefit from adapting foreign-developed technologies, and an undervalued exchange rate also is a factor. In this study, growth slowed from 5.6 to 2.1 percent per year. A drop of that magnitude in China’s growth would take it to the 6–7 percent range. Again, there is nothing magic about correlation, and China might escape the threshold by developing its own internal undeveloped “country” in western China.
Yet economics and politics will intersect. Both Chinese and foreign analysts have long understood the major “fault lines” (“adversities”) that might seriously affect China’s ability to sustain rapid economic growth.[9] How might these adversities occur, and by how much would they affect China’s growth? If growth slowed, what sectors and what people would be hit? How much would unemployment and underemployment increase, including for college graduates, which already is a problem? The available data are plentiful, from government numbers of protests to middle-class behavior. If those were continually laid against other sources, like Chinese bloggery and whatever hints were available from human intelligence or political reporting, again, the likelihood of definitive warning would be very low. But the process might suggest new places to look and open analysis to unsuspected correlations.
[1] This article draws on the concluding chapter of Wilhelm Agrell and Gregory F. Treverton, Beyond the Great Divide: Relevance and Uncertainty in National Intelligence and Science for Policy, forthcoming from Oxford University Press.
[2] U.S. Army, 1948, courtesy of Sir David Omand.
[3] The distinctions were not new with former Secretary of Defense Donald Rumsfeld, but he used them famously in a Pentagon press briefing, December 12, 2002. Known unknowns are what we know we don’t know, and known unknowns are what we don’t know we don’t know.
[4] For the shortcomings of a traditional intelligence approach in the face of social changes like the Arab spring, see Eyal Pascovich, “Intelligence Assessment Regarding Social Developments: The Israeli Experience,” International Journal of Intelligence and Counterintelligence, 26, no. 1 (2013): 84–114.
[5] This section draws on Gregory F. Treverton, “Assessing Threats to the U.S.,” in Great Decisions 2013 (New York: Foreign Policy Association, 2012), pp. 101ff.
[6] Henry S. Rowen, “Will China’s High Speed Ride Get Derailed?,” unpublished paper, 2011.
[7] Henry S. Rowen, “When Will the Chinese People Be Free?,” Journal of Democracy 18, no. 3 (2007): 38–52.
[8] Barry Eichengreen, Donghyun Park, and Kwanho Shin, “When Fast Growing Economies Slow Down: International Evidence and Implications for China,” National Bureau of Economic Research R Working Paper 16919(2011)., availabe at http://www.nber.org/papers/w16919.
[9] Charles Wolf et al., Fault Lines in China’s Economic Terrain, MR–1686–NA/SRF ed. (Santa Monica: RAND Corporation, 2003).